In the ongoing buzz following the launch of ChatGPT, people are once again predicting a near-future singularity, when artificial intelligence will match and then exceed human intelligence.
Author Erik J. Larson makes a strong case that this is a myth.
In his book “The Myth of Artificial Intelligence: Why Computers Can’t Think the Way We Do” Larson describes three levels of reasoning – deductive, inductive and abductive.
In deduction, there is a clear logical outcome. For example: All humans are mortal. Socrates is a human. Therefore, Socrates is mortal.
On the other hand, inference by induction comes from observing a sample of occurrences and drawing a conclusion. For example: Given that the large number of swans I have observed are all white, I conclude that all swans are probably white.
By contrast, abductive inference relies on postulating a hypothesis based on some limited set of information. For example, if we come across a car hood which is warm to touch, there could be several logical explanations – the car engine has recently operated, or the direct sun has warmed the bonnet, or the dog has been sleeping on the hood, or there is a fire under the hood, etc. A scan of the environment could point to the most likely, but not necessarily certain, explanation.
ChatGPT has demonstrated that AI is excellent at producing real-time answers when it can rapidly draw on a vast pool of information. It can, for example, combine a good grasp of natural language using transformer models, trawl its database for key information and then assemble it into a coherent essay at any word length you specify. This is indeed impressive.
However, the machine-learning behind AI-models like ChatGPT is fundamentally based on inductive inference, which allows them to recognize patterns within narrow, predefined tasks. Such models lack context, common sense, and genuine reasoning. They cannot make consistently sound autonomous decisions in complex, real-world scenarios; in truth, these models are only as effective as the data they’re trained on and can falter in unfamiliar situations. Humans can falter in unfamiliar situations too, but we have intuition and imagination. In the realm of abductive reasoning, AI is no match against humans – yet.
Larson suggests that instead of trying to replicate human intelligence, we should focus on creating tools that augment human capabilities. He advocates for a collaborative approach, where AI systems support and enhance human decision-making rather than attempting to replace it.
These ideas parallel those of Garry Kasparov, the former chess world champion, who coined Kasparov’s Law which posits that informed humans using AI well can outperform experts using AI badly. This should make sense to any of you who have interacted with AI models. The best results from an AI come with insightful prompting and contextualizing from you, the human user.
It follows therefore that it may be wiser to embrace the opportunity for a promotion (as Kasparov puts it) and learn how to make use of AI tools, rather than fret over the potential threats from AI.
Larson’s book is a critical examination of the current state and future potential of AI, and is a timely antidote to some of the panicky scenarios or inflated prospects being floated in the popular media.
Kasparov says it best:
“Machines have calculations. We have understanding. Machines have instructions. We have purpose. Machines have objectivity. We have passion. There is one thing that only a human can do. That’s to dream. So let us dream big.”
That is not to say that there won’t be disruptions in the job market, the professions and education. But I’m leaving that discussion for my future post, Survive and Thrive with AI.
The writer is a co-author of Court of the Grandchildren, a novel set in 2050s America.
Main image credit: Alexandra Koch via Pixabay
For posts on similar themes, consider:

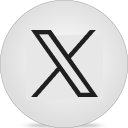



